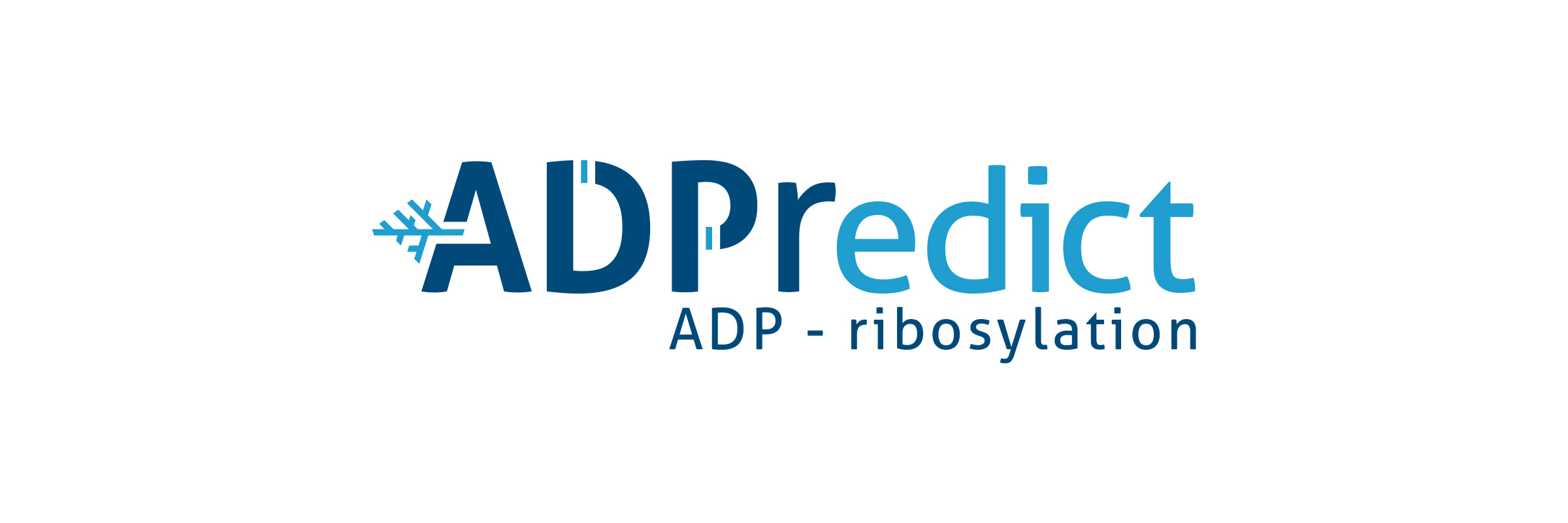
Web application for the prediction of ADP-ribosylated Glutamic and Aspartic residues
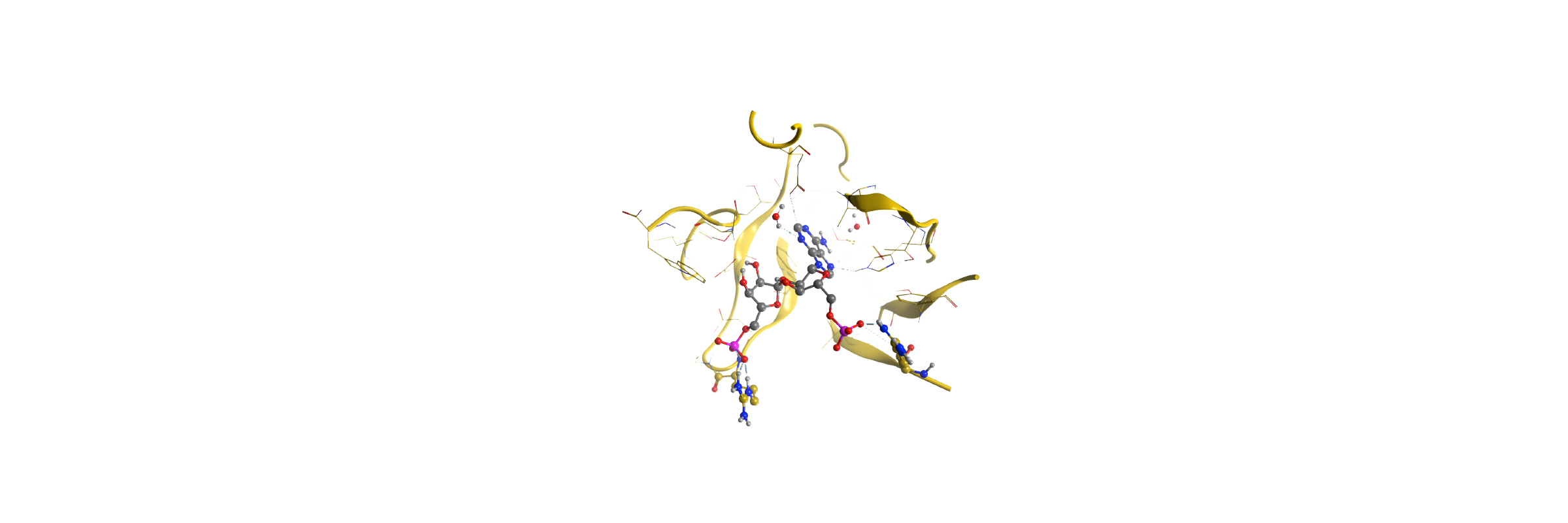
In-house homology model PARP12-iso-ADP-ribose complex detail
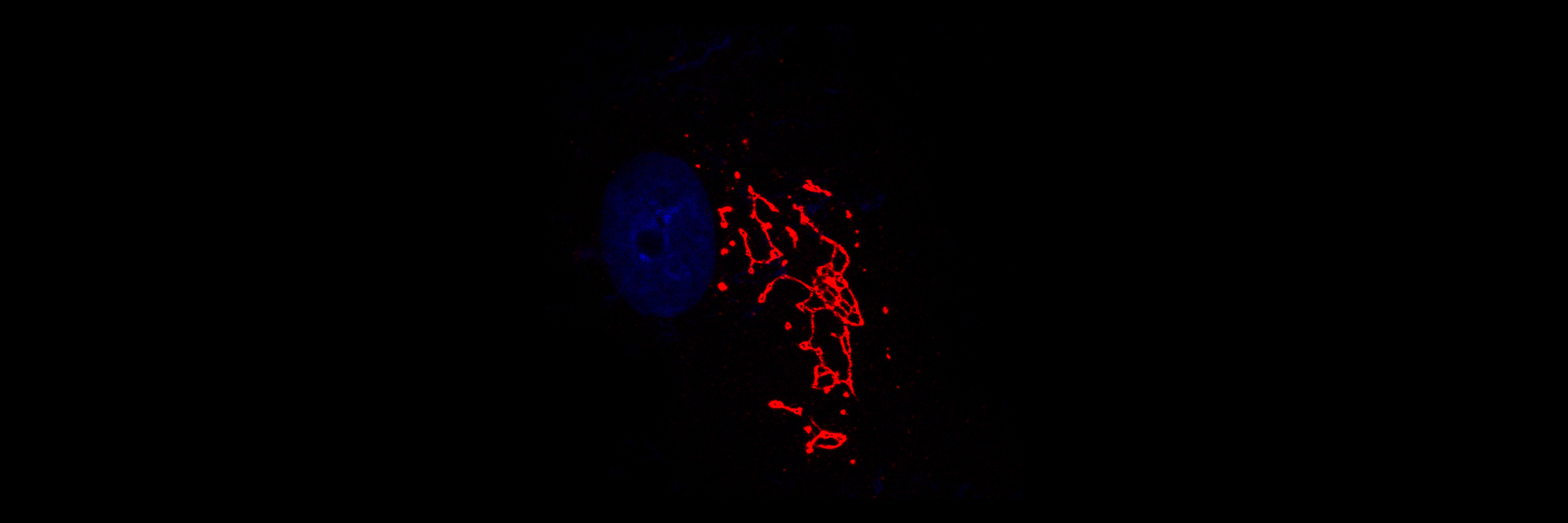
Golgi complex localisation of PARP12 (red) in human fibroblasts. Nucleus is stained with Hoechst (blue)
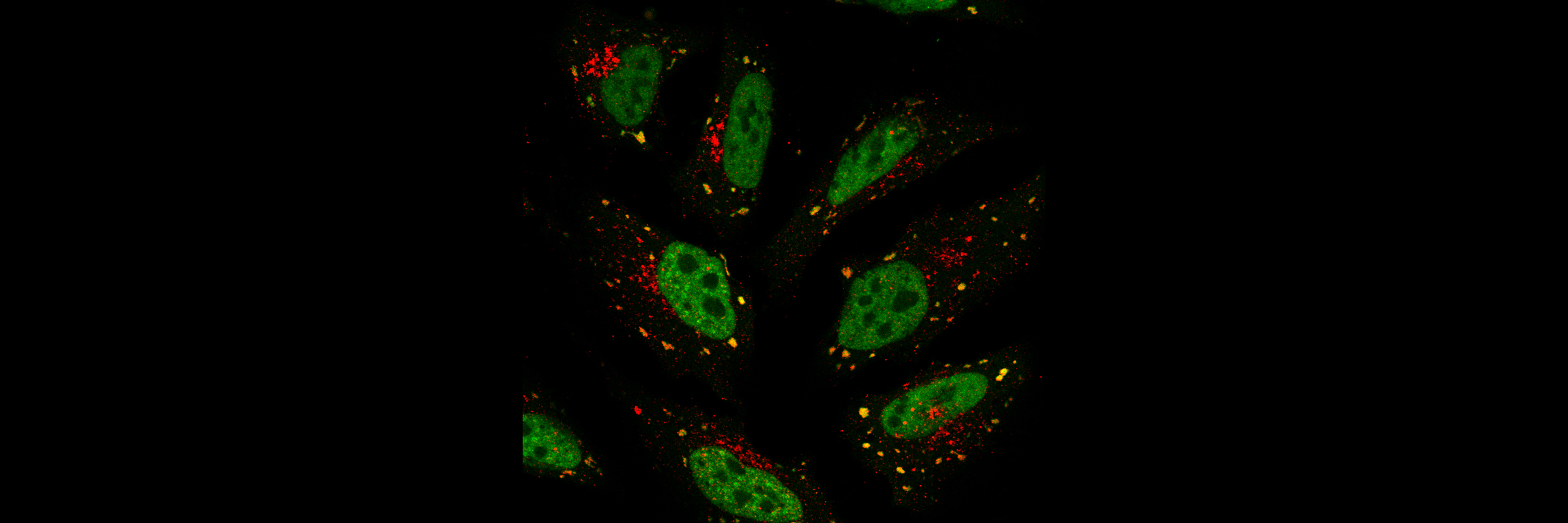
Stress granules induced by oxidative stress are labelled with FITC-conjugated oligod(T) (green) and PARP12 (red)
About
Post-translational Modifications
Post-translational modifications (PTM) exponentially increase the variety of protein functions in the organism, allowing a fine-tuned and rapid response to a wide range of stimuli occurring in both physiological and pathological conditions. Accounting for functional groups or small molecules covalent addition (such as phosphorylation or ubiquitination), rather than redox modifications, bonds formation or peptides cleavage (either degradative or activating), PTMs are numerous and highly diversified processes, governed by a number of enzymes. To date, while some of these events have been exhaustively described, for many others, among which ADP-ribosylation, the characterization of the actors involved as well as the cellular environment and the cascade of the events downstream are only partially understood.
ADP-ribosylation
ADP-ribosylation consists of the enzymatic transfer of ADP-ribose moiety (ADPr) from NAD+ to protein targets, and it is accompanied by the release of nicotinamide. This reaction is reversible and thus the extent of the protein modification by ADP-ribosylation depends on the activity of cellular ADP-ribosylhydrolases that reverse the reaction by hydrolysing the protein ADP-ribose linkage. This reaction can be operated by both ecto-enzymes (arginine specific ADP-ribosyltransferases) and intracellular enzymes, known as poly-ADP-ribosylpolymerases (PARPs)1,2. Enzymes of the PARP family are defined by the presence of a conserved catalytic domain3; mechanistically, they can add a single unit of ADPr (mono-ARTs) or multiple moieties to form long and branched ADPr polymers (PARPs) mostly on Lysine and acidic residues4,5. In human, there are 17 members of this family, having distinct structural domains, activities, localizations and functions1,6,7. Indeed, PARPs are involved in many different biological events, among which damaged DNA detecting and repairing machinery activation, cell survival, inflammation, transcriptional regulation, proteasome degradation, chromatin relaxation and stress response6. The involvement of ADP-ribosylation in key cell functions -often altered in cancer- led to an increased interest of the scientific community towards PARP enzymes, with the aim to unravel related molecular pathways, and thus open new fields for innovative cancer drug development
ADPredict
ADPredict is the first dedicated computational tool for the prediction of ADP-ribosylated acidic residues, freely accessible online (REF).
Based on i) physicochemical features, ii) in-house derived secondary structure related descriptors and iii) 3-Dimensional properties of a set of experimentally retrieved ADP-ribosylated proteins, ADPredict is developed using principal component analysis and machine learning techniques.
It offers of a selection of predictive models, proved to be effective and statistically robust via internal and external validation stages, as well as to perform better than the only predictor of ADP-ribosylation available online.
Designed to address and support molecular biology studies, ADPredict was thus developed in order to maximize the number of correctly predicted sites within the first top ranked positions, allowing the user to easily enter the experimental validation stages.
The authors firmly think that ADPredict represents a powerful forward thrust for the scientific research in the field.
References
1Grimaldi, G. et al. From toxins to mammalian enzymes: the diversity of mono-ADP-ribosylation. Front Biosci (Landmark Ed) 1985;54:73-100.
2Ueda, K. and Hayaishi, O. ADP-ribosylation. Annu Rev Biochem 2015;20:389-404.
3Otto, H., et al. In silico characterization of the family of PARP-like poly(ADP-ribosyl)transferases (pARTs). BMC Genomics 2005;6:139.
4Messner, S. et al. PARP1 ADP-ribosylates lysine residues of the core histone tails. Nucleic Acids Res 2010;38(19):6350-6362.
5Vyas, S. et al. Family-wide analysis of poly(ADP-ribose) polymerase activity. Nat Commun 2014;5:4426.
6Gupte, R. et al. PARPs and ADP-ribosylation: recent advances linking molecular functions to biological outcomes. Genes Dev 2017;31(2):101-126.
7Vyas, S. et al. A systematic analysis of the PARP protein family identifies new functions critical for cell physiology. Nat Commun 2013;4:2240.
Acknowledgements
The authors would like to thank Dr. Daniela Corda, Dr. Alberto Luini and their research groups for the helpful discussions that inspired and supported the development of this instrument.
ADPredict web server development, deployment and maintenance is curated by Nuovi S.O.C.I.
Version and Release
ADPredict 1.1, Jan, 2018.
Citing ADPredict
If you use ADPredict in your work, please cite:
Matteo Lo Monte, Candida Manelfi, Marica Gemei, Daniela Corda, Andrea Rosario Beccari; ADPredict: ADP-ribosylation site prediction based on physicochemical and structural descriptors, Bioinformatics, Volume 34, Issue 15, 1 August 2018, Pages 2566–2574, https://doi.org/10.1093/bioinformatics/bty159
Features
Data
A refined collection of 1018 experimentally derived unique ADP-ribosylated sites (Glutamic and Aspartic residues), referring to 317 human proteins, was used as training set for the development of predictive algorithms1. For 54 proteins one or more reference resolved structures were finely selected among often a plurality, so allowing the calculation of secondary structure related and 3-D properties as well for 135 of these ADP-ribosylated sites.
Features
Tree classes of properties were taken into account for the description of the training set: i) the physicochemical properties of the amino acids as described by the principal components of Z-Scales, ST-Scales, Prot-FP and MSWHIM descriptors2; ii) the secondary structure motifs of the sub-sequences as encoded with an in-house generated hashing strategy; iii) the 3-D properties as calculated on the whole-protein structure
These features were extracted from a progressively wider sub-sequence (from 5 to 33 amino acids), centered on the key residue, for both the ADP-ribosylated sites, the true positives, and the remaining acidic residues not reported to be modified, and so considered as true negatives.
For the physicochemical and 3-D properties, the principal components explaining the 75 % of the variance were used as descriptors for models development; the secondary structure based descriptor was, instead, generated form the ratio of the frequency of a given hashed motif in TPs and TNs entries.
Models
Recursive Partitioning Tree (RP), Random Forests (RF) and Support Vector Machines (SVM) methods were used in parallel for models development, performing the calculations for each variable length sub-sequences (VLS)
A leave-one-out strategy was exploited to evaluate and select the best performing models for each descriptors, using the Enrichment Factor3 (EF) and Receiving Operating Characteristic4 (ROC) as evaluation functions. A consensus model, accounting for the mean prediction of all the selected models, resulted to outperform each of them, collecting their contribution into a single predictive model, ADPredict.
Validation
A stability assessment of the selected models was performed with an intensive boot-strapping approach, consisting of 1K runs of leave-10%-out t, iteratively selecting training and test sets with an in-house developed routine for the random number selection, so avoiding any sampling bias.
Two additional datasets of experimentally retrieved ADP-ribosylated sites, published by Kraus5 and Nielsen6 teams, containing 1150 and 1137 entries, respectively, were used as external validation.
A successful benchmarking session was undertaken comparing the predictive power of ADPredict that of the only predictor of ADP-ribosylation available online, ModPred7, in foreseeing all the three experimental datasets.
Web Service
ADPredict is a freely accessible web application. The ADPredict website is implemented using LAMP, which allows to provide a fluent and responsive user experience in displaying and handling the output data, calculated on the fly in a completely automated Pipeline Pilot workflow. It runs on CentOS 7, Apache/2.4.6, MariaDB-5.5.52, PHP/5.4.16.
References
1Zhang, Y. et al. Site-specific characterization of the Asp- and Glu-ADP-ribosylated proteome. Nat Methods 2013;10(10):981-984.
2van Westen, G.J. et al. Benchmarking of protein descriptor sets in proteochemometric modeling (part 1): comparative study of 13 amino acid descriptor sets. J Cheminform 2013;5(1):41..
3Kirchmair, J. et al. Evaluation of the performance of 3D virtual screening protocols: RMSD comparisons, enrichment assessments, and decoy selection--what can we learn from earlier mistakes? J Comput Aided Mol Des 2008;22(3-4):213-228.
4Fawcett. An introduction to ROC analysis. Pattern Recognition Letters - Special issue: ROC analysis in pattern recognition 2006;861-874.
5Gibson, B.A. et al. Chemical genetic discovery of PARP targets reveals a role for PARP-1 in transcription elongation. Science 2016;;7:12917..
6Martello, R. et al. Proteome-wide identification of the endogenous ADP-ribosylome of mammalian cells and tissue. Nat Commun 2016;7:12917.
7Pejaver, V. et al. The structural and functional signatures of proteins that undergo multiple events of post-translational modification. Protein Science 2014;23(8):1077-1093.
Tutorial
Input
The user can perform the search by entering the UniProt entry or the UniProt entry nameof the protein of interest, as well as by entering a custom sequence in Fasta format (only standard amino acids are allowed). The query entry will be supported by an autocomplete function, so avoiding typos or incorrected searches.
Output
As a first feedback, ADPredict reports in table the count of all Glutamic and Aspartic residues present in the protein primary sequence and the related information:
- the amino acidic position within the sequence
- the VLS in which it is located (VLS 11 is preferred, otherwise VLS 9, if lower the site is marked as discarded and not predicted)
- the relative secondary structure string (if available)
- the selected resolved structure (PDB ID) (if available)
For custom query, only AAD-based predictive models will be calculated.
Sub-sequences in the table are hyper-linked with the related page on the UniProtKn database (uniprot.org), as well as clicking on the PDB ID leads to the related entry of the RCSB Protein Data Bank (rcsb.org), both open in a new tab.
Beside the table, a pie chart summarizes the count of residues of interest, the information they come with and the models for the calculation of which they are available.
Submitting the query leads to the predictions output, represented by both a plot and a table, completely interactive and available for the download.
The web server by default will calculate the ADPredict model as well as the other five models described above, three accounting for the physicochemical properties, one for secondary structure and one for the 3-D features.
The computational time required for a prediction task depends on the availability of the resolved structure, and, then, on the number of models that have to be calculated, ranging from few tens of seconds up to a couple of minutes.
Video
Work in progress...
Contacts
Matteo Lo Monte, m.lomonte@ibp.cnr.it
Candida Manelfi, candida.manelfi@dompe.com
Andrea Rosario Beccari, andrea.beccari@dompe.com
Joint Bioinformatics Group (JBG), Institute of Protein Biochemistry (IBP), National Research Council, Naples, Via Pietro Castellino 111, 80131, Italy.
Phone: +39.081.6132.339
Website: www.adpredict.net